Machine Learning in Education: Transforming Classrooms through Technology
Machine learning in education represents an advanced application of artificial intelligence and machine learning technology to enhance the educational process. Machine learning allows for personalized education, tailoring the learning experience to each student's individual needs and pace. It enables the delivery of interactive educational materials and customized content that motivates students and enhances their comprehension. This transformation in classrooms opens the door to leveraging modern technologies such as deep learning and data analysis to provide rich and inspiring learning experiences.
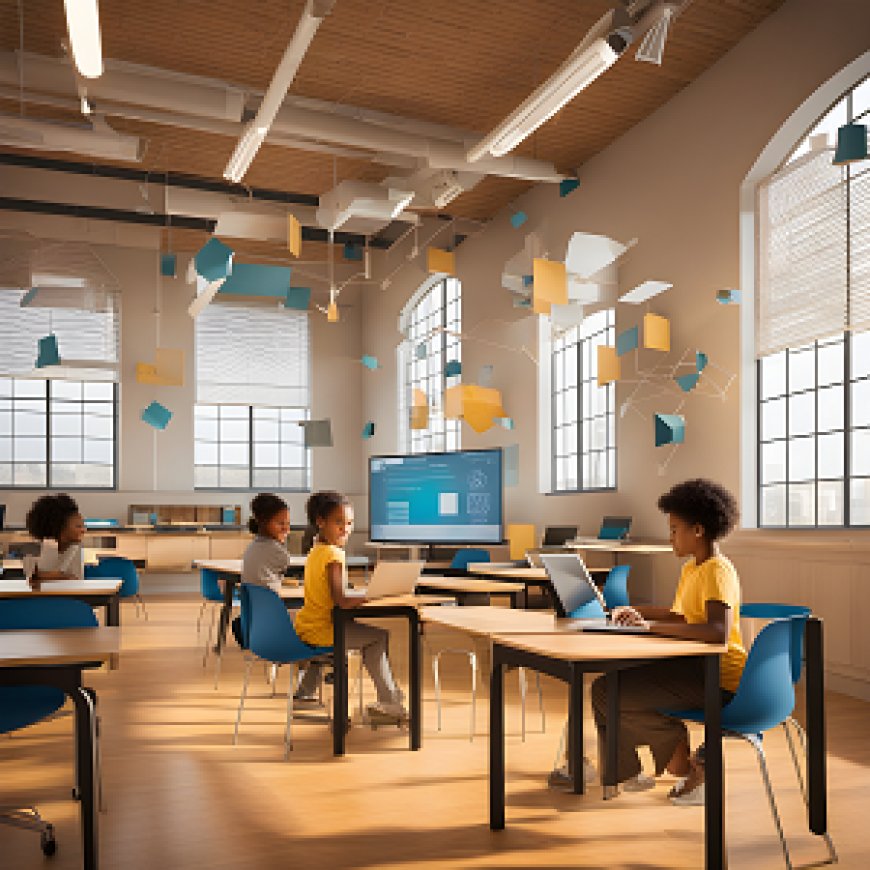
Machine learning in the field of education represents a revolutionary advancement that combines the concepts of artificial intelligence and technology to transform and enhance the processes of learning and teaching. This concept is pivotal in improving the quality and efficiency of classrooms, allowing education to be personalized according to each individual's pace and specific needs. In this article, we will explore how machine learning in education can make a breakthrough in the field of learning through advanced technology that enhances student interaction and provides tailored educational materials for each learner. These transformations open new doors for us to leverage modern techniques like deep learning and data analysis to deliver rich and inspiring educational experiences.
The Role of Machine Learning in Enhancing the Learning Experience
Machine learning in education, commonly referred to as educational machine learning, has become a pivotal technology in transforming and enhancing the learning experience. This innovative approach leverages the power of algorithms, data analysis, and automation to facilitate personalized and adaptive learning for students at various levels of education. The integration of machine learning in education has introduced significant improvements in the way students are taught and assessed, and it has the potential to revolutionize the entire educational system.
Personalized Learning Paths:
- Machine learning algorithms play a crucial role in tailoring educational content to individual students' needs. By analyzing students' past performance and preferences, these algorithms can generate personalized learning paths. This ensures that students receive content that is appropriately challenging and aligned with their interests, which can significantly enhance their motivation and engagement with the material.
Predictive Analytics:
- Machine learning models are adept at predicting students' future performance based on their past behavior. By examining historical data such as test scores, attendance, and participation, these models can provide educators with early warnings about students who may be at risk of falling behind. This proactive approach allows teachers to intervene and provide additional support to struggling students, ultimately improving their learning outcomes.
Automated Grading and Feedback:
- Machine learning can streamline the grading process by automating the evaluation of assignments, quizzes, and exams. This not only saves educators a significant amount of time but also ensures consistency in grading. Additionally, machine learning can provide instant feedback to students, allowing them to identify and address their weaknesses promptly, which can enhance the learning experience and foster a sense of self-directed learning.
Adaptive Content Delivery:
- One of the most significant contributions of machine learning in education is the ability to adapt content delivery in real-time. These algorithms can adjust the difficulty and pacing of learning materials to match the student's current level of understanding. This adaptive approach ensures that students are neither overwhelmed nor bored, resulting in a more efficient and effective learning process.
Data-Driven Decision-Making:
- Educational institutions can benefit from the insights derived from machine learning models. By analyzing data from various sources, including student performance, attendance, and engagement, schools and universities can make data-driven decisions to improve their teaching methodologies, resource allocation, and curriculum design. This, in turn, enhances the overall learning experience for all students.
Intelligent Tutoring Systems:
- Machine learning-powered intelligent tutoring systems offer students an interactive and personalized learning experience. These systems can respond to students' queries, offer explanations, and guide them through the learning material, mimicking the experience of one-on-one tutoring. Such systems have the potential to increase student success rates and foster a deeper understanding of the subject matter.
Continuous Improvement:
- Machine learning in education is a dynamic field that continuously evolves to adapt to the changing needs of students and educators. As more data is collected and analyzed, machine learning models become increasingly accurate and sophisticated, leading to ongoing enhancements in the learning experience.
machine learning in education is a transformative force that plays a critical role in enhancing the learning experience. Its ability to personalize learning paths, predict student performance, automate grading, and offer adaptive content delivery makes it a valuable tool for educators and students alike.
Challenges and Opportunities of Machine Learning in Education
Challenges and opportunities abound in the realm of machine learning in education. As educational institutions and organizations increasingly adopt this innovative technology, it is imperative to scrutinize the complexities associated with its implementation, as well as the potential benefits it offers.
Challenges:
-
Data Privacy and Security: One of the foremost challenges in deploying machine learning in education is the need to safeguard sensitive student data. Educational institutions collect vast amounts of personal information, and ensuring the privacy and security of this data is paramount. This requires stringent data protection measures and compliance with relevant regulations, such as the Family Educational Rights and Privacy Act (FERPA) in the United States.
-
Ethical Concerns: Machine learning algorithms may inadvertently reinforce biases present in historical data, leading to potential discrimination or unfair treatment. Bias mitigation and algorithm fairness are critical challenges, and ensuring that machine learning models do not perpetuate or amplify existing inequalities is a top priority.
-
Accessibility: Integrating machine learning tools and technologies into education systems can pose accessibility challenges. Not all students have equal access to devices or the internet, potentially leaving some students at a disadvantage. Addressing these accessibility issues is essential to ensure equitable educational opportunities.
-
Teacher Training: Teachers and educators need to be proficient in using machine learning tools effectively. Training and upskilling are essential to harness the full potential of these technologies. Providing educators with the necessary resources and training programs is a considerable undertaking.
-
Cost: Implementing machine learning solutions can be costly, and many educational institutions may face budget constraints. The procurement of appropriate hardware, software, and the hiring of skilled personnel can strain financial resources.
Opportunities:
-
Personalized Learning: Machine learning enables personalized learning experiences, catering to individual student needs. These technologies can adapt content and pacing, ensuring that each student progresses at their own optimal rate, resulting in improved engagement and performance.
-
Early Intervention: Machine learning models can predict when a student is at risk of falling behind, allowing educators to intervene early and provide targeted support. This proactive approach enhances student success rates and prevents learning gaps.
-
Automated Assessment: Automated grading and feedback systems powered by machine learning streamline the assessment process. This saves educators time and offers immediate feedback to students, promoting self-directed learning and improvement.
-
Curriculum Enhancement: Machine learning can analyze educational data to identify areas where curricula need improvement. This data-driven approach helps in curriculum development, making it more effective and relevant for students.
-
Efficient Resource Allocation: Data analysis through machine learning can help educational institutions optimize resource allocation, ensuring that students have access to the best tools, materials, and learning experiences.
-
Enhanced Research and Development: Machine learning can support research in education by analyzing large datasets and identifying trends and patterns in student performance, helping researchers to develop evidence-based educational practices.
machine learning in education presents both challenges and opportunities. While data privacy, ethics, and accessibility concerns must be addressed, the potential benefits, including personalized learning, early intervention, and curriculum enhancement, make machine learning a compelling technology for improving the educational experience.
Practical Applications of Machine Learning in the Classroom
Practical applications of machine learning in the classroom are increasingly prevalent, reflecting the broader integration of technology in the educational sector. Machine learning, with its capacity for data analysis and predictive modeling, holds the potential to revolutionize the learning environment.
-
Personalized Learning:
Machine learning algorithms are utilized to craft personalized learning experiences. These algorithms analyze students' data and learning patterns, enabling the tailoring of educational content to individual needs. Such personalized learning paths ensure that students are engaged with content that matches their learning pace and preferences, contributing to more effective learning outcomes.
-
Adaptive Content Delivery:
One of the key practical applications of machine learning in the classroom is adaptive content delivery. Machine learning models adjust the difficulty and pacing of educational materials based on students' progress. This adaptation ensures that students are not overwhelmed or under-challenged, fostering a more efficient learning process.
-
Automated Grading and Feedback:
Machine learning automates the grading process, making it faster and more consistent. Additionally, it enables instant feedback to students, aiding in their comprehension and learning improvement. Educators are thus relieved of the arduous task of manual grading, allowing them to dedicate more time to teaching and mentoring.
-
Early Intervention and Predictive Analytics:
Machine learning models are employed for early intervention through predictive analytics. By analyzing historical data, these models can predict when a student might struggle or fall behind. This allows educators to identify students in need of additional support and tailor their interventions, potentially preventing learning gaps.
-
Curriculum Enhancement:
Machine learning can assess the effectiveness of existing curricula by analyzing data on student performance and engagement. This data-driven approach helps educational institutions adapt and improve their curricula to align more closely with student needs and expectations.
-
Intelligent Tutoring Systems:
Machine learning can create intelligent tutoring systems that provide students with personalized guidance and support. These systems can offer instant explanations, answer questions, and adapt to students' learning progress. This mimics the experience of one-on-one tutoring, which can lead to deeper understanding and improved learning outcomes.
-
Data-Driven Decision-Making:
Educational institutions can utilize machine learning to make data-driven decisions. By analyzing a plethora of data points, ranging from student performance to resource allocation, schools and universities can optimize their operations, ultimately leading to an enhanced learning experience.
-
Classroom Management and Student Engagement:
Machine learning can help educators manage classrooms more efficiently. For instance, it can provide insights into student engagement and attendance patterns, enabling teachers to adjust their teaching methods and support students more effectively.
practical applications of machine learning in the classroom are multifaceted, offering a broad array of benefits for students and educators alike. Personalized learning, adaptive content delivery, automated grading, and early intervention are among the key advantages. Furthermore, machine learning facilitates data-driven decision-making, allowing educational institutions to continuously improve their teaching methodologies and curriculum, ensuring an enhanced and more effective learning experience.
How Can Artificial Intelligence Improve Educational Personalization?
Artificial Intelligence (AI) holds the promise of significantly enhancing educational personalization through its applications, particularly machine learning, within the realm of education. Educational institutions, educators, and ed-tech companies are increasingly exploring AI-driven solutions to cater to the unique needs of students.
-
Student Profiling and Learning Analytics:
Machine learning algorithms can analyze a wealth of student data, including past academic performance, learning preferences, and strengths and weaknesses. By processing this data, AI can create detailed student profiles, offering a comprehensive understanding of each student's learning style and capabilities. This allows for tailored educational experiences that consider individual needs.
-
Adaptive Content Delivery:
AI-powered systems can customize learning materials based on each student's progress and preferences. For example, if a student excels in a certain subject, the system can adjust the curriculum to provide more challenging content. Conversely, if a student struggles, it can offer additional support and simpler materials. This adaptive approach ensures that students receive content suited to their specific skill levels.
-
Personalized Learning Paths:
Machine learning algorithms can create personalized learning paths for students. These paths adapt to the individual's pace, helping them advance at an optimal rate. This ensures that no student is left behind or held back, promoting a balanced and effective learning experience.
-
Early Intervention and Predictive Analytics:
AI can predict when a student is likely to face academic challenges or fall behind. By analyzing historical data and learning patterns, machine learning models can provide educators with early warning signals, enabling them to intervene proactively and provide targeted support. This approach minimizes learning gaps and increases student success rates.
-
Automated Assessment and Feedback:
AI systems can automate the assessment process, including grading assignments, tests, and quizzes. This not only saves educators time but also offers students immediate feedback on their performance. Instant feedback fosters self-directed learning, as students can identify their mistakes and take steps to improve.
-
Intelligent Tutoring Systems:
Intelligent tutoring systems, powered by AI and machine learning, provide students with personalized guidance and support. These systems can answer questions, provide explanations, and adapt to a student's learning progress, mimicking the experience of one-on-one tutoring.
-
Data-Driven Decision-Making:
Educational institutions can use AI and machine learning to make data-driven decisions, such as resource allocation and curriculum development. By analyzing a wide range of educational data, institutions can optimize their operations and create a more personalized learning environment.
-
Curriculum Enhancement:
Machine learning models can analyze data on student performance to identify areas where curricula need improvement. This data-driven approach helps educational institutions refine their curriculum to be more effective and relevant.
artificial intelligence, especially machine learning, has the potential to significantly enhance educational personalization. By leveraging data analysis, adaptive content delivery, and personalized learning paths, AI can create tailored educational experiences for each student. Additionally, early intervention, automated assessment, and data-driven decision-making contribute to a more effective and personalized learning environment, ensuring that students receive the support and resources they need to succeed.
Machine Learning: Guiding the Way to the Future
Machine learning, particularly when applied within the educational context, is considered a critical tool guiding the way to the future of education. This technology is driving substantial advancements in the education sector, transforming traditional teaching and learning methods.
-
Personalized Learning:
Machine learning is revolutionizing education by enabling personalized learning experiences. It accomplishes this through data analysis and predictive modeling. By examining student data, machine learning algorithms can tailor learning materials and experiences to meet individual students' unique needs. This personalization ensures that students receive content that matches their learning pace, style, and interests.
-
Adaptive Content Delivery:
One of the primary applications of machine learning in education is the delivery of adaptive content. Machine learning models adjust the difficulty and pacing of educational materials based on individual student progress. This adaptability prevents students from feeling overwhelmed or unchallenged, thus facilitating a more efficient learning process.
-
Automated Assessment and Grading:
Machine learning streamlines the assessment process. It automates grading, making it quicker and more consistent. Additionally, it provides instant feedback to students, aiding their comprehension and learning improvement. Educators are consequently freed from the time-consuming task of manual grading, allowing them to focus more on teaching.
-
Early Intervention and Predictive Analytics:
Machine learning models are employed to predict when a student may face learning difficulties or fall behind. Through the analysis of historical data and learning patterns, these models can provide early indicators, allowing educators to intervene and provide targeted support. This proactive approach reduces the occurrence of learning gaps and increases student success rates.
-
Enhanced Curriculum Development:
Machine learning can analyze educational data to determine areas of improvement within curricula. By understanding student performance and engagement, educational institutions can refine and develop more effective educational programs that better suit students' needs.
-
Intelligent Tutoring Systems:
Machine learning enables the creation of intelligent tutoring systems that offer personalized guidance and support to students. These systems provide immediate explanations, answer questions, and adapt to students' learning progress, emulating the experience of one-on-one tutoring.
-
Data-Driven Decision-Making:
Educational institutions are utilizing machine learning for data-driven decision-making. By analyzing a wide array of data, such as student performance and resource allocation, schools and universities can optimize their operations, ultimately leading to an improved learning experience.
-
Resource Allocation Efficiency:
Machine learning helps educational institutions efficiently allocate resources. Data analysis through machine learning aids institutions in understanding the needs and performance of students, ensuring that they have access to the best tools, materials, and learning experiences.
machine learning is unequivocally guiding the way to the future of education. Its potential for personalized learning, adaptive content delivery, automated assessment, and early intervention cannot be understated. Furthermore, the data-driven decision-making and efficient resource allocation contribute to a more effective and enhanced learning experience for students. Machine learning, particularly in the context of education, is poised to reshape the way we teach and learn, offering a glimpse into an exciting future of education.
The Impact of Machine Learning on Curriculum Development
Machine learning, when harnessed for curriculum development in the field of education, is undeniably impacting and reshaping the educational landscape. The incorporation of machine learning into curriculum development processes has introduced a level of precision and adaptability that was previously unattainable.
-
Data-Driven Curriculum Design:
Machine learning leverages vast sets of educational data to inform and improve curriculum design. Through the analysis of historical student performance, learning patterns, and preferences, machine learning algorithms identify areas of strength and weakness. This data-driven approach assists in the creation of curricula that cater to the specific needs and learning styles of students.
-
Personalization and Customization:
Machine learning allows for the personalization of curricula on an unprecedented scale. By processing individual student data, machine learning models can develop customized learning paths. These paths are tailored to accommodate students' unique learning paces and requirements, ensuring that they receive content suited to their current skill levels and interests.
-
Adaptive Content Delivery:
One of the fundamental contributions of machine learning to curriculum development is adaptive content delivery. Machine learning systems continuously monitor student progress and adapt the difficulty and pace of content accordingly. This adaptability ensures that students are neither overwhelmed by overly challenging material nor held back by content that is too simple.
-
Optimizing Resource Allocation:
Machine learning is employed to optimize resource allocation within educational institutions. By analyzing data on student engagement, attendance, and performance, schools and universities can make informed decisions regarding the allocation of resources such as faculty, materials, and technology. This data-driven approach helps maximize the efficiency of resource utilization.
-
Early Intervention and Support:
Machine learning models can predict when a student is likely to face academic challenges or fall behind. By analyzing historical data and learning patterns, these models provide educators with early warning signals, enabling them to intervene proactively and provide targeted support. Early intervention minimizes learning gaps and enhances student success rates.
-
Automated Curriculum Updates:
Curriculum development is an ongoing process, and machine learning streamlines the task of keeping curricula current and relevant. Machine learning models can analyze educational trends and changing needs, helping educational institutions make timely updates to their curricula.
-
Enhanced Content Recommendations:
Machine learning algorithms can offer content recommendations to students and educators. Based on a student's past performance and preferences, the system can suggest supplementary materials, resources, or courses. This feature encourages self-directed learning and allows educators to tailor content to individual student needs.
-
Continuous Improvement and Feedback Loop:
Machine learning enables a continuous feedback loop in curriculum development. Data from student performance and outcomes are analyzed to identify areas in need of improvement. This data-driven feedback informs iterative curriculum enhancements, ensuring that educational content remains effective and up-to-date.
machine learning is profoundly impacting curriculum development in education. By providing data-driven insights, personalization, and adaptability, it is enhancing the quality of education. Furthermore, its ability to optimize resource allocation, provide early intervention, and facilitate continuous improvement ensures that curricula are responsive to the ever-evolving needs of students and educational institutions. Machine learning's influence on curriculum development is a testament to its potential to revolutionize and improve the field of education.
Enhancing Performance Assessment through Machine Learning
Performance assessment in education is a critical component for evaluating the effectiveness of teaching methods and the progress of students. It provides valuable insights into the strengths and weaknesses of educational strategies, helping institutions make data-driven decisions. Machine learning, when applied to performance assessment in education, offers a range of benefits and opportunities, revolutionizing the way educational assessment is conducted.
-
Data-Driven Performance Metrics:
Machine learning introduces data-driven performance metrics that provide a more comprehensive understanding of student achievements. By analyzing a wide range of data, including test scores, attendance, and engagement, machine learning algorithms generate insights that go beyond traditional grading methods. This approach enables educators to identify trends, patterns, and areas in need of improvement.
-
Automated Grading and Feedback:
Machine learning streamlines the grading process by automating it. It can assess and grade assignments, quizzes, and tests quickly and consistently. Moreover, it offers instant feedback to students, pointing out their mistakes and areas for improvement. This immediate feedback fosters a better understanding of the material and supports student learning.
-
Personalized Assessment:
One of the remarkable features of machine learning is its ability to personalize assessment methods. It can adapt assessment criteria based on individual student characteristics, learning styles, and progress. This ensures that students are evaluated fairly and in a way that accommodates their unique needs.
-
Early Warning Systems:
Machine learning models can predict when a student may be at risk of falling behind or encountering difficulties. By analyzing historical data and comparing a student's current performance, these models provide early warning signals. Educators can use this information to intervene and provide targeted support, reducing the likelihood of students falling behind.
-
Adaptive Testing:
Machine learning enables the creation of adaptive tests that adjust the difficulty level of questions based on a student's responses. This ensures that students are appropriately challenged, resulting in a more accurate evaluation of their knowledge and skills.
-
Resource Allocation Optimization:
Machine learning is used to optimize the allocation of educational resources. By analyzing data on student performance and engagement, educational institutions can make informed decisions regarding faculty allocation, materials, and technology. This optimization ensures that resources are directed where they are most needed.
-
Longitudinal Performance Tracking:
Machine learning enables the longitudinal tracking of student performance over time. This allows educators to monitor individual progress, identify trends, and assess the long-term impact of their teaching methods.
-
Enhanced Predictive Analytics:
Machine learning enhances predictive analytics for performance assessment. By considering a wide array of variables, including socio-economic factors, learning environments, and student demographics, it can predict not only a student's current performance but also their future success or challenges.
machine learning is revolutionizing performance assessment in education by introducing data-driven, automated, and personalized methods. It allows for early intervention, adaptive testing, and optimized resource allocation. Through predictive analytics and the longitudinal tracking of performance, machine learning offers educators and institutions a more comprehensive and accurate view of student progress and educational effectiveness. The application of machine learning in performance assessment is a significant step toward improving the quality of education.
Distance Learning and Machine Learning: The Future of Education
Distance learning, in combination with machine learning, has become an integral part of the modern educational landscape, revolutionizing the way education is delivered and received. This article delves into the intricacies of this educational evolution, emphasizing the role of machine learning in education.
-
Overview of Distance Learning:
Distance learning, often referred to as online education or e-learning, involves the delivery of educational content and instruction to students who are geographically separated from their educators. This mode of learning has gained immense popularity, especially in recent years, owing to advancements in technology and increased internet accessibility.
-
Machine Learning in Education:
Machine learning, a subset of artificial intelligence, has found a significant place in the field of education. It revolves around the development of algorithms and models that enable computers to analyze and make predictions based on data. In the context of education, machine learning is used to personalize learning experiences, automate administrative tasks, and enhance educational outcomes.
-
Personalization of Learning:
One of the most remarkable applications of machine learning in education is the personalization of learning experiences. Machine learning algorithms analyze students' learning patterns, preferences, and performance to tailor educational content and pace to individual needs. This ensures that students receive content suited to their skill levels and learning styles, leading to improved engagement and retention.
-
Automated Assessment and Feedback:
Machine learning has streamlined the assessment process in distance learning. It can automatically grade assignments, quizzes, and tests, providing instant feedback to students. This automated grading system reduces the workload on educators and ensures students receive feedback promptly, facilitating a deeper understanding of the material.
-
Data-Driven Decision-Making:
Machine learning leverages educational data to inform decision-making processes. It enables institutions to identify trends, predict outcomes, and optimize resource allocation. This data-driven approach helps in the effective management of educational resources and the continuous improvement of online courses.
-
Early Intervention and Support:
Machine learning models can predict when a student is likely to face academic challenges or fall behind in online courses. By analyzing data, these models provide educators with early warning signals, allowing them to intervene proactively and provide targeted support, thus reducing dropout rates and improving student success.
-
Adaptive Content Delivery:
Machine learning ensures that educational content is delivered adaptively. It continuously monitors student progress and adjusts the difficulty and pace of content to match their abilities, preventing students from becoming overwhelmed or bored with the material.
-
Resource Optimization:
Machine learning optimizes the allocation of resources in distance learning. It helps educational institutions allocate faculty, materials, and technology efficiently by analyzing data on student engagement, attendance, and performance.
-
Predictive Analytics for Student Success:
Machine learning models can predict student success or identify potential challenges based on a wide range of variables, including socio-economic factors, learning behaviors, and demographics. This proactive approach allows educators to provide targeted support, enhancing overall student outcomes.
-
Continuous Improvement:
Machine learning facilitates a continuous improvement loop in distance learning. Educational data is analyzed to identify areas in need of enhancement, which informs iterative course updates and improvements, ensuring that online courses remain effective and up-to-date.
distance learning, when coupled with machine learning, represents the future of education. The personalization, automation, data-driven decision-making, early intervention, and adaptive content delivery made possible by machine learning have the potential to reshape and enhance the educational experience for students across the globe. As technology continues to evolve, the synergy between distance learning and machine learning will play a pivotal role in the ongoing transformation of education.
Digital Transformation: How Schools Can Embrace Machine Learning
Digital transformation is an evolving process in the realm of education. It involves the integration of digital technologies to improve educational methods, administrative processes, and overall learning experiences. Within the context of digital transformation, machine learning has emerged as a critical component, particularly in schools and educational institutions.
-
Understanding Digital Transformation:
Digital transformation in education is a comprehensive shift from traditional methods towards digital technologies. It encompasses a wide range of initiatives, including the incorporation of digital tools, online resources, and data-driven decision-making. The ultimate goal is to enhance the efficiency and effectiveness of educational processes.
-
Machine Learning in Education:
Machine learning, a subset of artificial intelligence, is a technology that empowers computers to analyze data, recognize patterns, and make predictions. In education, machine learning is leveraged to personalize learning experiences, streamline administrative tasks, and offer data-driven insights into student performance.
-
Personalization of Learning:
One of the central roles of machine learning in digital transformation is the personalization of learning experiences. Machine learning algorithms analyze students' preferences, learning styles, and performance to tailor educational content and delivery to individual needs. This individualized approach ensures that each student receives materials that match their skill levels and learning preferences, leading to increased engagement and improved learning outcomes.
-
Automated Administrative Tasks:
Machine learning streamlines administrative tasks in schools. It can automate admissions, scheduling, and even financial management. This automation reduces the workload on administrative staff, allowing them to focus on more strategic aspects of school management.
-
Data-Driven Decision-Making:
Machine learning enhances data-driven decision-making. By analyzing a wealth of educational data, schools can gain insights into student progress, curriculum effectiveness, and resource allocation. This data-driven approach supports effective resource management and continuous improvement of educational offerings.
-
Early Intervention and Support:
Machine learning models can predict when a student may be at risk of academic challenges. By identifying at-risk students early, schools can provide proactive support, reducing dropout rates and ensuring the success of a wider range of students.
-
Adaptive Learning Platforms:
Machine learning powers adaptive learning platforms, which adjust the difficulty and pace of content based on individual student performance. This ensures that students are adequately challenged and can progress at their own rates, maximizing their learning potential.
-
Resource Allocation Optimization:
Machine learning helps schools optimize the allocation of resources, from faculty assignments to educational materials. By analyzing data on student engagement and performance, institutions can allocate resources where they are needed most, improving overall efficiency.
-
Predictive Analytics for Student Success:
Machine learning can predict student success or identify potential challenges based on a wide range of variables, such as socio-economic factors and learning behaviors. This proactive approach enables educators to provide targeted support, improving student outcomes.
-
Continuous Improvement:
Machine learning contributes to a cycle of continuous improvement in schools. Data analysis identifies areas that need enhancement, leading to iterative updates and improvements in curriculum and teaching methods.
the integration of machine learning into the digital transformation of schools is pivotal for enhancing the educational experience. It enables personalization, automation, data-driven decision-making, early intervention, and adaptive learning. As schools continue to adapt to the digital age, the symbiotic relationship between digital transformation and machine learning will play a vital role in shaping the future of education, empowering educators and improving student outcomes.
Ethical and Challenges Surrounding Machine Learning in Education
Ethical considerations and challenges in the realm of machine learning in education represent a critical facet of this evolving landscape. Machine learning applications in educational settings have garnered significant attention, but they also raise numerous ethical dilemmas and challenges that must be carefully addressed. In this comprehensive analysis, we delve into the intricacies of these ethical concerns and challenges related to the use of machine learning in education.
Ethical Considerations:
-
Privacy Concerns: Privacy is a paramount concern in the context of machine learning in education. Collecting and analyzing student data for personalized learning can potentially infringe upon individual privacy rights. It is crucial for educational institutions and technology providers to implement robust data protection measures and obtain informed consent from students and their guardians.
-
Data Security: The security of educational data is a significant ethical challenge. Sensitive information, including academic records and personal data, must be safeguarded against breaches or unauthorized access. The responsibility of securely managing this data falls on educational institutions and technology vendors.
-
Algorithmic Bias: Machine learning algorithms are trained on historical data, which may contain biases. These biases can perpetuate inequalities and stereotypes in education. It is vital to regularly audit algorithms to identify and rectify biases to ensure fair and equitable treatment of all students.
-
Transparency and Explainability: Machine learning models are often complex and not easily interpretable. This lack of transparency poses challenges in explaining the reasoning behind automated decisions, such as grading or content recommendations. Ethical considerations demand efforts to enhance transparency and ensure stakeholders understand the decision-making process.
Challenges:
-
Limited Access to Technology: Inequities in technology access among students can exacerbate educational disparities. Machine learning systems often require access to computers and the internet. Ensuring that all students have equal access is a critical challenge.
-
Teacher Training: Integrating machine learning into the educational process necessitates teacher training. Many educators may lack the necessary skills to effectively use and manage machine learning systems. Institutions must invest in training programs to bridge this gap.
-
Resource Allocation: Implementing machine learning in education demands significant resources, both in terms of technology infrastructure and personnel. Budget constraints and resource allocation are challenges institutions need to address.
-
Data Quality: The success of machine learning models is contingent on data quality. Incomplete, inaccurate, or biased data can hinder the effectiveness of these systems. Maintaining high-quality educational data is a continuous challenge.
-
Evaluation and Accountability: Assessing the impact and effectiveness of machine learning in education is a complex task. Schools and institutions must develop methods for evaluating the outcomes and accountability of these systems to ensure they are meeting educational goals.
-
Student and Teacher Acceptance: The successful implementation of machine learning in education depends on the acceptance and buy-in from both students and teachers. Resistance to change or concerns about job displacement can be challenging to address.
-
Legal and Regulatory Compliance: Meeting legal and regulatory requirements in education, including data protection laws, adds another layer of complexity. Compliance with these laws while harnessing the potential of machine learning is an ongoing challenge.
-
Overreliance on Technology: There is a concern that overreliance on machine learning may diminish the role of human educators. Striking a balance between technological assistance and human guidance is a continuous challenge.
while machine learning holds immense promise for improving education, it also brings forth a set of ethical considerations and challenges. Privacy, bias, data security, and transparency must be carefully managed. Moreover, addressing issues related to access, teacher training, resource allocation, and data quality is pivotal for the successful implementation of machine learning in education. A thorough understanding of these ethical and challenges is essential for harnessing the full potential of machine learning in education while maintaining ethical standards and ensuring equitable access to quality education.
In conclusion
machine learning in education is a dynamic field that promises to reshape the way we teach and learn. As technology continues to evolve, the potential for leveraging machine learning in education is boundless. It offers us the opportunity to provide personalized and adaptive learning experiences, identify areas where students may need additional support, and enhance the overall quality of education. However, it is important to strike a balance between technological innovation and the human element in education. While machine learning can automate and optimize many aspects of teaching and learning, it cannot replace the vital role of educators in guiding and mentoring students. The future of education is undoubtedly intertwined with machine learning, and as we navigate this transformation, it is crucial to keep the learner's growth and development at the heart of our educational endeavors, ensuring that technology serves as a powerful tool to augment the learning experience.
What's Your Reaction?
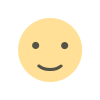
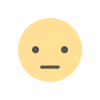
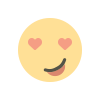
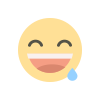
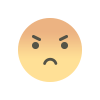
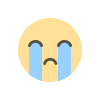
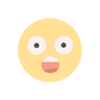