Big Data Analysis Using Artificial Intelligence: Methods and Applications
Analyzing big data using artificial intelligence is a field that has witnessed tremendous growth in our current era. Combining big data analytics techniques with the power of artificial intelligence allows us to utilize information in ways that surpass traditional methods. This approach relies on leveraging machine learning algorithms and artificial neural networks to extract patterns and provide precise recommendations. Its applications range from improving businesses and facilitating strategic decision-making to delivering better healthcare and gaining a deeper understanding of consumer behavior. Analyzing big data with artificial intelligence is a promising field that is positively transforming the world.
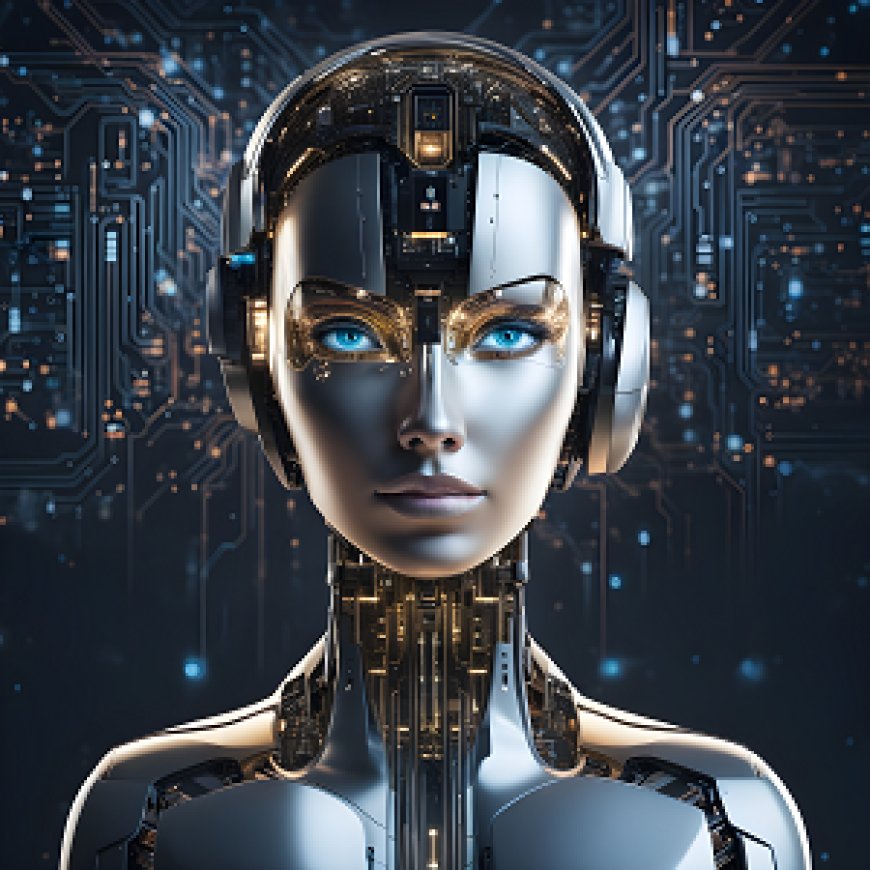
In our current era, the analysis of big data using artificial intelligence is a field that is experiencing remarkable growth. This approach combines big data analytics techniques with the power of artificial intelligence to enable us to harness information in ways that go beyond traditional methods. It relies on the use of machine learning algorithms and artificial neural networks to extract patterns and provide precise recommendations. Its applications include improving businesses, facilitating strategic decision-making, enhancing healthcare, and gaining deeper insights into consumer behavior. Big data analysis through artificial intelligence is a promising field that is bringing about a positive transformation in our world.
The Role of Artificial Intelligence in Empowering Big Data Analysis
Artificial Intelligence (AI) plays a pivotal role in the realm of big data analysis. The synergy between AI and big data has the potential to revolutionize how we collect, process, and extract insights from vast and complex datasets.
1. Data Collection and Management:
AI contributes significantly to big data analysis by automating data collection and management processes. AI-driven tools can gather, ingest, and clean large volumes of data from various sources, such as sensors, social media, and IoT devices. This automation improves data quality and consistency.
2. Data Preprocessing and Cleansing:
Big data often arrives in raw and unstructured forms. AI techniques, like natural language processing and computer vision, help preprocess and cleanse the data by organizing it, removing duplicates, and handling missing values. This step is crucial for ensuring that the data is ready for analysis.
3. Data Integration and Fusion:
AI algorithms facilitate the integration of heterogeneous data sources. They can align data from different formats and standards, providing a unified view of the information. This integration is essential for drawing meaningful insights from a diverse range of data.
4. Real-time Data Processing:
Big data analysis demands real-time processing capabilities. AI-powered streaming analytics can process incoming data as it is generated, allowing organizations to react to changing conditions and trends promptly. This is particularly valuable for industries like finance and e-commerce.
5. Pattern Recognition and Anomaly Detection:
AI excels at recognizing patterns and anomalies within large datasets. Machine learning models can identify trends, correlations, and outliers, making it easier to extract valuable insights and detect irregularities that may signify fraud, defects, or security breaches.
6. Predictive Analytics:
AI models enable predictive analytics by using historical data to forecast future trends and outcomes. Businesses can leverage this capability for demand forecasting, risk assessment, and decision-making, enhancing their strategic planning processes.
7. Natural Language Processing (NLP):
NLP is instrumental in analyzing unstructured text data, such as customer reviews, social media comments, and news articles. AI-powered sentiment analysis and text summarization tools assist in deriving meaningful insights from textual content.
8. Machine Learning Algorithms:
Machine learning algorithms, a subset of AI, are indispensable in big data analysis. They can classify, cluster, and regress data to uncover hidden patterns and relationships within the data, facilitating informed decision-making.
9. Deep Learning for Image and Speech Analysis:
In scenarios involving image and speech data, deep learning techniques shine. Convolutional neural networks (CNNs) excel in image analysis, while recurrent neural networks (RNNs) are adept at processing sequential data, such as speech and time series data.
10. Data Visualization:
AI-powered data visualization tools create meaningful, intuitive representations of complex data. These visualizations simplify the communication of insights to stakeholders, aiding in the decision-making process.
11. Scalability and Efficiency:
AI can handle big data's immense volume and complexity with efficiency. It enables parallel processing and can scale horizontally to accommodate increasing data volumes, making it well-suited for the big data landscape.
12. Continuous Learning and Adaptation:
AI models can learn and adapt over time. They can automatically adjust to changing data patterns, making them suitable for dynamic environments where the data distribution evolves.
13. Reducing Human Bias:
AI can reduce human bias in data analysis by providing impartial, data-driven insights. This is particularly important in fields such as healthcare and finance, where biased decisions can have serious consequences.
artificial intelligence is a driving force in the realm of big data analysis. It contributes to data collection, preprocessing, integration, and real-time processing. It excels in pattern recognition, predictive analytics, and natural language processing, while machine learning and deep learning models uncover hidden insights. AI enhances scalability, efficiency, and adaptability, making it an indispensable tool in the era of big data. By harnessing the power of AI, organizations can extract valuable knowledge from vast and complex datasets, ultimately enabling more informed decision-making and driving innovation.
Advancements in Big Data Analysis Techniques with Artificial Intelligence
Advancements in the field of big data analysis techniques, especially when coupled with artificial intelligence, have ushered in a new era of data-driven decision-making. The marriage of big data analysis and artificial intelligence (AI) offers unprecedented opportunities to extract valuable insights from vast and complex datasets.
1. Machine Learning Algorithms:
Machine learning algorithms have significantly evolved to accommodate the intricacies of big data analysis. Traditional statistical methods are often inadequate to handle the sheer volume and variety of big data. Machine learning models, including decision trees, support vector machines, and deep learning neural networks, have shown great promise in extracting patterns and relationships from large datasets. These algorithms can classify, cluster, and predict data, making them indispensable tools for uncovering hidden insights.
2. Scalable Infrastructure:
Big data analysis requires scalable infrastructure to store, process, and analyze massive datasets. The advancements in distributed computing frameworks, such as Apache Hadoop and Spark, have empowered organizations to process data in parallel across clusters of computers. These technologies offer enhanced data processing capabilities, enabling the analysis of vast datasets in a more efficient and cost-effective manner.
3. Real-time Data Processing:
The ability to process and analyze data in real-time is crucial in today's fast-paced business environment. Advancements in stream processing frameworks like Apache Kafka and Flink have made it possible to analyze incoming data as it's generated. This real-time analysis enables businesses to make immediate decisions based on the most up-to-date information.
4. Natural Language Processing (NLP):
NLP has witnessed significant advancements, especially in sentiment analysis, named entity recognition, and text summarization. AI-powered NLP tools are invaluable in analyzing vast volumes of unstructured text data, such as social media posts, customer reviews, and news articles. These techniques assist in deriving meaningful insights from textual content.
5. Deep Learning for Image and Speech Analysis:
Deep learning, a subset of machine learning, has revolutionized image and speech analysis. Convolutional neural networks (CNNs) excel in image recognition and analysis, while recurrent neural networks (RNNs) are adept at processing sequential data, such as speech and time series data. These advancements have paved the way for improved image and speech recognition applications.
6. Enhanced Data Integration:
Big data often comprises data from diverse sources in varying formats. Advancements in data integration techniques have made it easier to harmonize and unify this heterogeneous data. Techniques like data wrangling and data virtualization enable organizations to create a single, cohesive view of the data, facilitating more accurate and holistic analysis.
7. Automated Feature Engineering:
Feature engineering is a critical aspect of data analysis. Automating this process has become more sophisticated, thanks to AI-driven techniques. Automated feature engineering tools can identify relevant features within the data, reducing the time and effort required for feature selection and extraction.
8. Advanced Data Visualization:
Effective data visualization is key to understanding and conveying the insights derived from big data analysis. AI-driven data visualization tools are capable of creating intricate, interactive visualizations that provide stakeholders with a more intuitive and comprehensive view of the data. These tools are instrumental in making the insights more accessible and actionable.
9. Prescriptive Analytics:
Beyond descriptive and predictive analytics, advancements in big data analysis techniques have ushered in the era of prescriptive analytics. These techniques provide actionable recommendations based on historical data, enabling organizations to make informed decisions and optimize processes.
10. Ethical Considerations:
With the increasing reliance on AI and big data analysis, ethical considerations have come to the forefront. Advancements in the field include the development of ethical AI frameworks and tools to ensure that data analysis is conducted responsibly and without biases.
the advancements in big data analysis techniques, when coupled with artificial intelligence, have transformed the way organizations leverage data for decision-making. Machine learning algorithms, scalable infrastructure, real-time data processing, NLP, and deep learning have significantly enhanced the capabilities of big data analysis. The ability to integrate diverse data sources, automate feature engineering, and create advanced data visualizations makes the insights derived from big data more accessible and actionable. As we move forward, ethical considerations will continue to play a vital role in ensuring that big data analysis with AI is conducted in a responsible and unbiased manner.
Benefits of Big Data Analysis Using Artificial Intelligence
The benefits of employing big data analysis in conjunction with artificial intelligence (AI) are numerous and significant.
1. Improved Decision-Making:
One of the primary benefits of big data analysis with AI is the enhancement of decision-making processes. The ability to analyze massive datasets in real-time enables organizations to make informed and timely decisions. AI algorithms can uncover hidden patterns and relationships within the data, offering valuable insights that human analysts might overlook.
2. Enhanced Data Accuracy:
Big data analysis, when coupled with AI, can significantly improve data accuracy. AI-driven data cleaning and validation processes can identify and rectify errors, redundancies, and inconsistencies in the data. This results in higher data quality and more reliable analysis outcomes.
3. Predictive Analytics:
Big data analysis, powered by AI, enables organizations to leverage predictive analytics. AI models can identify trends and make forecasts based on historical data. This capability is invaluable for anticipating market trends, customer preferences, and future events.
4. Real-Time Insights:
With the integration of AI, big data analysis can provide real-time insights. This is particularly beneficial for businesses operating in dynamic environments where immediate action is required. Real-time data analysis allows organizations to react swiftly to changing conditions and capitalize on emerging opportunities.
5. Cost Reduction:
Big data analysis with AI can lead to cost reductions in various ways. By automating data processing and analysis, organizations can lower operational costs and improve efficiency. AI can also identify cost-saving opportunities, such as optimizing supply chains or reducing energy consumption.
6. Personalized Customer Experiences:
AI-driven big data analysis enables businesses to create personalized customer experiences. By analyzing customer data and behavior, organizations can tailor their products, services, and marketing strategies to individual preferences, leading to increased customer satisfaction and loyalty.
7. Fraud Detection and Prevention:
AI is highly effective in identifying fraudulent activities. Big data analysis with AI can analyze vast volumes of transaction data to detect unusual patterns or anomalies that may indicate fraud. This is crucial for financial institutions and e-commerce businesses.
8. Competitive Advantage:
Organizations that leverage big data analysis and AI gain a competitive edge. They can respond to market shifts faster, optimize their operations, and offer innovative solutions and services based on data-driven insights. This positions them ahead of competitors who rely on traditional approaches.
9. Improved Healthcare and Research: I
n the healthcare sector, big data analysis with AI has revolutionized patient care and medical research. AI can analyze medical records, clinical data, and genomic information to provide accurate diagnoses and treatment recommendations. It also accelerates drug discovery and clinical trials.
10. Scalability:
Big data analysis with AI is highly scalable. It can handle ever-increasing volumes of data without significant infrastructure upgrades. This scalability is crucial in the era of exploding data volumes.
11. Data-Driven Innovation:
AI-driven big data analysis encourages data-driven innovation. Organizations can uncover new business opportunities, product ideas, and process improvements by exploring data insights. This fosters a culture of innovation and continuous improvement.
12. Risk Management:
AI and big data analysis contribute to effective risk management. By analyzing historical and real-time data, organizations can assess and mitigate risks more proactively. This is particularly important in industries such as insurance and finance.
the benefits of employing big data analysis with artificial intelligence are extensive and touch various aspects of business and decision-making. From improved decision-making and enhanced data accuracy to real-time insights and cost reduction, these technologies offer organizations the tools needed to thrive in today's data-driven world. The advantages span across industries, from personalized customer experiences in retail to improved healthcare in the medical field. Leveraging big data analysis with AI is not only a competitive advantage but a key driver of innovation and efficiency in the modern business landscape.
Challenges and Complexities of Big Data Analysis and How Artificial Intelligence Helps
Analyzing big data presents a plethora of challenges and complexities that organizations must navigate in their quest for actionable insights. This painstakingly detailed exploration delves into the intricacies of big data analysis and how artificial intelligence (AI) plays a pivotal role in overcoming these challenges.
1. Data Volume and Velocity:
Big data is characterized by its immense volume and the speed at which it is generated. Traditional data processing tools struggle to handle this scale, leading to performance bottlenecks. AI-driven algorithms are capable of processing and analyzing data in real-time, making them instrumental in dealing with the rapid influx of data.
2. Data Variety:
Big data comes in diverse formats, from structured databases to unstructured text and multimedia. AI, particularly natural language processing (NLP) and computer vision, enables the extraction of valuable insights from these varied data types. This versatility is essential for comprehensive analysis.
3. Data Quality:
Ensuring data quality is an ongoing challenge in big data analysis. Dirty or incomplete data can lead to inaccurate results. AI can assist in data cleaning and validation, automatically identifying and rectifying errors, enhancing data accuracy.
4. Data Security and Privacy:
The vast amount of data being analyzed raises concerns about security and privacy. Protecting sensitive information is paramount. AI algorithms can help in identifying and mitigating security threats and ensuring data compliance with privacy regulations.
5. Scalability:
As data continues to grow, organizations require scalable solutions. AI and cloud computing offer the scalability needed to handle increasing data volumes without significant infrastructure upgrades.
6. Interpretability:
AI models, especially deep learning models, are often considered black boxes, making it challenging to interpret their decisions. Interpretable AI models are crucial for ensuring transparency and trust in big data analysis.
7. Bias and Fairness:
AI systems may inadvertently introduce bias into analyses, which can lead to unfair outcomes. Detecting and mitigating bias in AI algorithms is a complex challenge, particularly in the context of big data.
8. Data Integration:
Combining data from various sources is another intricate task. AI tools can assist in data integration, harmonizing different datasets, and facilitating a unified view for analysis.
9. Cost:
Implementing AI in big data analysis can be expensive, requiring specialized talent and infrastructure. Organizations need to weigh the benefits against the costs and develop cost-effective strategies.
10. Skill Gap:
Finding and retaining professionals with the required AI and big data expertise is a constant challenge. Upskilling the workforce and fostering a culture of data literacy is essential.
11. Regulatory Compliance:
Adhering to data protection and privacy regulations is a growing concern. AI tools can aid in ensuring regulatory compliance by automating data governance processes.
12. Model Maintenance:
AI models used for big data analysis require continuous monitoring and maintenance to remain effective. This adds complexity to the analytical process.
13. Real-Time Analysis:
In today's fast-paced world, real-time analysis is crucial. AI enables real-time data processing and decision-making, but it comes with its own set of technical complexities.
14. Ethical Considerations:
The ethical use of AI in big data analysis is of paramount importance. Ensuring fairness, transparency, and accountability in AI systems is an ongoing challenge.
15. Data Storage and Retrieval:
Storing and retrieving massive amounts of data for analysis can be resource-intensive. AI-driven data management solutions can optimize data storage and retrieval processes.
the complexities and challenges of big data analysis are multifaceted, encompassing issues related to data volume, variety, quality, security, and more. Artificial intelligence plays a critical role in addressing these challenges, offering solutions for real-time analysis, bias detection, and scalability. Nevertheless, organizations must remain vigilant in ensuring ethical, secure, and compliant practices throughout the big data analysis journey. Balancing the advantages of AI-driven big data analysis with the complexities and challenges it presents is an ongoing imperative for modern businesses.
The Social and Economic Impact of Big Data Analysis by Artificial Intelligence
The social and economic impact of big data analysis by artificial intelligence (AI) is a subject of great significance. It encompasses a wide range of implications for society.
1. Data-Driven Decision-Making:
One of the key social impacts of big data analysis with AI is the transformation of decision-making processes. Businesses, governments, and organizations now rely on data-driven insights to make informed choices. This shift has the potential to improve efficiency and effectiveness across various sectors.
2. Personalized Experiences:
Big data analysis powered by AI enables a high degree of personalization. Social platforms, e-commerce, and entertainment services utilize data-driven recommendations to tailor content and offerings to individual preferences, enhancing user experiences.
3. Healthcare Advancements:
The healthcare sector benefits from AI-driven big data analysis in numerous ways. Medical research and diagnosis are significantly improved with the ability to process and analyze vast volumes of patient data. This results in more accurate diagnoses and personalized treatment plans.
4. Economic Growth:
Big data analysis fosters innovation and economic growth. It helps businesses identify market trends, optimize supply chains, and develop new products and services. This, in turn, leads to increased competitiveness and job creation.
5. Enhanced Customer Service:
AI-driven big data analysis allows businesses to provide better customer service. Chatbots and virtual assistants use data to understand customer inquiries and respond effectively, improving customer satisfaction.
6. Fraud Detection:
AI plays a vital role in fraud detection. Financial institutions and online platforms use AI algorithms to analyze transactions and identify anomalies that may indicate fraudulent activity, safeguarding both individuals and businesses.
7. Social Media Insights:
Social media platforms use big data analysis and AI to understand user behavior and preferences. This information is valuable for targeted advertising, content optimization, and trend prediction.
8. Resource Optimization:
Across industries, resource optimization is facilitated by big data analysis. AI helps manage resources efficiently, reducing waste and environmental impact.
9. Challenges and Privacy Concerns:
The social impact is not without its challenges. Big data analysis and AI raise concerns about data privacy and security. Balancing the benefits with individual privacy rights remains a complex issue.
10. Job Displacement and Reskilling:
Economically, the automation of tasks through AI-driven big data analysis can lead to job displacement. However, it also creates a demand for new skill sets, necessitating workforce reskilling.
11. Ethical Considerations:
The ethical use of AI and big data analysis is a critical issue. Ensuring fairness, transparency, and accountability in decision-making processes is an ongoing concern.
12. Accessibility Divide:
The economic impact of big data analysis by AI can create disparities. Access to advanced analytics tools and technologies may not be equitable, leading to an accessibility divide.
13. Regulatory Frameworks:
Governments are adapting and developing regulatory frameworks to address the social and economic implications of big data analysis and AI. These regulations aim to ensure responsible data use and protect consumers' rights.
14. Market Disruption:
Industries are experiencing disruption as businesses harness the power of big data and AI. Incumbent players face the challenge of adapting to this rapidly evolving landscape.
the social and economic impact of big data analysis by artificial intelligence is profound. It revolutionizes decision-making, personalizes experiences, drives economic growth, and enhances various aspects of society. However, it also brings challenges related to privacy, job displacement, and ethical considerations. Striking the right balance between harnessing the benefits and addressing the challenges is crucial for realizing the full potential of big data analysis in the age of artificial intelligence.
Big Data Analysis and Artificial Intelligence in Healthcare
Big data analysis and artificial intelligence (AI) have become pivotal components in the healthcare sector, profoundly impacting various facets of patient care, clinical research, and healthcare management. The convergence of big data analysis and AI has introduced transformative changes in the healthcare landscape, enhancing decision-making processes, improving diagnostic accuracy, and facilitating personalized treatment strategies.
1. Improved Diagnosis and Predictive Analytics:
Big data analysis enables healthcare providers to harness vast datasets, encompassing patient records, medical imaging, and genomic information. AI algorithms can mine this data to identify patterns, anomalies, and correlations that may be imperceptible to human clinicians. This, in turn, leads to more accurate diagnoses and the early detection of diseases, ultimately saving lives.
2. Personalized Treatment Plans:
Tailoring treatment plans to individual patients is a fundamental aspect of modern healthcare, and big data analysis powered by AI plays a central role in achieving this. By considering a patient's genetic profile, medical history, and other relevant data, AI can recommend treatment strategies that are precisely suited to their unique needs.
3. Drug Discovery and Development:
Big data analytics and AI have expedited the drug discovery process. Researchers can analyze massive datasets to identify potential drug candidates, predict their efficacy, and even simulate their effects on the human body. This accelerates the development of new medicines and therapies.
4. Disease Surveillance and Outbreak Prediction:
The combination of big data and AI enables healthcare authorities to monitor and predict disease outbreaks. By analyzing data from various sources, including social media and healthcare records, AI can detect early signs of epidemics, allowing for proactive intervention and resource allocation.
5. Operational Efficiency:
Hospitals and healthcare institutions benefit from big data analysis and AI by optimizing their operational processes. These technologies can help streamline resource allocation, patient flow, and staff management, ultimately improving the quality of care and reducing costs.
6. Remote Monitoring and Telemedicine:
Big data analysis, in conjunction with AI, enables remote patient monitoring and telemedicine. Patients can transmit data from wearable devices or home monitoring systems, which AI can analyze to provide real-time insights to healthcare providers. This is especially valuable for managing chronic conditions and ensuring timely interventions.
7. Challenges and Privacy Concerns:
Despite the tremendous potential, the implementation of big data analysis and AI in healthcare also brings challenges. Privacy concerns and the security of sensitive medical data remain paramount, necessitating stringent regulations and safeguards.
8. Ethical Considerations:
The ethical use of AI in healthcare is a critical consideration. Ensuring that AI algorithms and decision-making processes are transparent, fair, and accountable is of utmost importance.
9. Regulatory Compliance:
Healthcare organizations must adhere to stringent regulatory frameworks when implementing big data analysis and AI. Compliance with laws like the Health Insurance Portability and Accountability Act (HIPAA) is essential to protect patient privacy.
10. Education and Training:
Healthcare professionals require education and training to effectively use AI tools and interpret the insights provided by big data analysis. Bridging the knowledge gap is an ongoing challenge.
the integration of big data analysis and artificial intelligence in healthcare has ushered in a new era of medical practice. It enhances diagnosis, treatment, and disease surveillance, ultimately improving patient outcomes. However, this transformative power also brings ethical and regulatory challenges that healthcare organizations must navigate as they embrace these technologies.
Security and Data Protection in Big Data Analysis with Artificial Intelligence
Security and data protection in big data analysis with artificial intelligence (AI) is of paramount importance, given the sensitive and vast nature of the datasets involved. The fusion of big data and AI has revolutionized data analysis and decision-making processes, but it has also introduced significant security concerns and privacy considerations.
1. Data Encryption:
Data encryption is a foundational security measure. In the realm of big data analysis, sensitive healthcare records, financial transactions, and personal information are regularly processed. Implementing robust encryption mechanisms ensures that data remains confidential and protected from unauthorized access.
2. Access Control:
Proper access control is indispensable in safeguarding data. It involves assigning permissions and restrictions to individuals or systems based on their roles and responsibilities. In big data analysis, only authorized personnel should be able to access, modify, or analyze the data.
3. Secure Data Transfer:
Data is often transmitted between systems for analysis. Ensuring the security of this data during transit is crucial. Secure protocols and encryption methods are used to protect data while it's in motion.
4. Data Masking and Anonymization:
Data masking and anonymization techniques are applied to protect sensitive information. By disguising or removing personally identifiable information, the privacy of individuals is preserved while still allowing for analysis.
5. Regular Auditing and Monitoring:
Continuous auditing and monitoring of data access and activities are vital for early threat detection. Suspicious behavior or unauthorized access attempts can be identified promptly, minimizing potential security breaches.
6. Secure Cloud Solutions:
Many big data analysis platforms leverage cloud infrastructure. Using reputable and secure cloud solutions, such as AWS or Azure, can enhance data security. Cloud providers offer built-in security measures and compliance certifications.
7. Compliance with Regulations:
Regulatory compliance is essential, especially in sectors like healthcare and finance. Adhering to standards like HIPAA and GDPR ensures that data is processed in accordance with the law and industry-specific regulations.
8. Threat Detection and Response:
Employing advanced threat detection systems is imperative. AI can be used not only for data analysis but also for identifying abnormal patterns that might indicate security threats. Rapid response and mitigation plans are essential.
9. Data Governance Policies:
Organizations must establish comprehensive data governance policies. These policies define data ownership, access procedures, and retention schedules. Clear guidelines help in maintaining data integrity and security.
10. Employee Training:
Human error is a significant security risk. Employees should undergo regular training on data security best practices, including recognizing phishing attempts and the responsible use of data.
11. Secure Data Storage:
Data storage solutions must meet strict security standards. Secure servers and storage systems prevent data breaches and unauthorized access.
12. Data Backup and Recovery:
Regular data backups are essential to mitigate data loss risks. Effective data recovery plans ensure that valuable information is not permanently lost in case of unforeseen events.
13. Privacy Impact Assessments:
Privacy impact assessments help in identifying and addressing potential privacy risks. Conducting these assessments is critical in designing data analysis projects that respect individuals' privacy.
security and data protection in big data analysis with artificial intelligence are multifaceted and meticulous processes. To fully harness the potential of big data analysis, organizations must prioritize security measures that encompass encryption, access control, monitoring, and compliance with regulations. AI, while a powerful tool for data analysis, also plays a role in identifying and mitigating security threats. By adopting these comprehensive security practices, organizations can enjoy the benefits of big data analysis while safeguarding sensitive information and ensuring data privacy.
Big Data Analysis and Its Applications in Marketing and Decision-Making
Big data analysis has become an integral component of modern marketing and decision-making processes. Its applications in these domains are vast and continue to expand, driven by the need to harness the enormous volumes of data generated in today's digital age. Let's delve into the intricacies of big data analysis and its applications in marketing and decision-making:
1. Data Collection and Aggregation:
The first step in big data analysis for marketing and decision-making is data collection. Companies gather data from various sources, including customer interactions, social media, web activity, and transaction records. This data is aggregated to create a comprehensive dataset for analysis.
2. Data Cleansing and Preprocessing:
Raw data often contains errors and inconsistencies. Before analysis, data must be cleansed and preprocessed. This involves removing duplicates, handling missing values, and ensuring data quality to obtain accurate results.
3. Segmentation and Customer Profiling:
One of the primary applications of big data analysis in marketing is customer segmentation. By analyzing customer data, companies can group customers with similar behaviors, preferences, and characteristics. These segments help in targeted marketing campaigns and product recommendations.
4. Predictive Analytics:
Big data analysis enables predictive modeling. By examining historical data, companies can predict future trends and customer behavior. This assists in making informed decisions regarding product development, pricing, and marketing strategies.
5. Personalization:
Personalized marketing is a key trend, and big data analysis is at its core. Companies use data to create customized experiences for customers, tailoring content, recommendations, and offers based on individual preferences.
6. Customer Journey Mapping:
Analyzing the customer journey is crucial for understanding how customers interact with a brand. Big data analysis helps in mapping these journeys, identifying touchpoints, and optimizing the customer experience.
7. Real-Time Analytics:
In today's fast-paced business environment, real-time analytics are essential. Big data analysis allows companies to make immediate decisions based on live data, such as tracking social media trends or website traffic.
8. A/B Testing and Optimization:
Marketing campaigns are often subjected to A/B testing. Big data analysis aids in analyzing the performance of different variations and optimizing campaigns for better results.
9. Market Basket Analysis:
Retailers use market basket analysis to uncover associations between products. By analyzing transaction data, they can determine which products are often purchased together and use this information for strategic product placement and promotions.
10. Sentiment Analysis:
Companies monitor social media and online reviews to gauge customer sentiment. Big data analysis allows sentiment analysis, providing insights into customer satisfaction and areas needing improvement.
11. Risk Management and Decision Support:
Beyond marketing, big data analysis is essential for overall decision support. It helps businesses assess risks, make strategic decisions, and adapt to market changes.
12. Data Visualization and Reporting:
To make data-driven decisions, clear data visualization and reporting are essential. Big data analysis tools often include dashboards and reports that simplify complex data into actionable insights.
13. Machine Learning and AI:
Machine learning and artificial intelligence are increasingly integrated into big data analysis for predictive analytics, customer recommendations, and automation of marketing tasks.
14. Regulatory Compliance:
In the context of data privacy regulations like GDPR, big data analysis also involves ensuring compliance in data handling and storage.
15. Continuous Improvement:
Big data analysis is an iterative process. Companies regularly refine their data analysis methods and strategies to adapt to evolving market dynamics.
big data analysis is a critical asset in modern marketing and decision-making. Its applications are vast, ranging from customer segmentation and predictive analytics to personalized marketing and risk management. With the continuous growth of data sources and advances in data analysis tools, its significance in these domains will only continue to expand. The ability to extract valuable insights from the ever-increasing volume of data is paramount for staying competitive in the business world.
Industries and Sectors Benefiting from Big Data Analysis Using Artificial Intelligence
Various industries and sectors are experiencing significant benefits from the integration of big data analysis with artificial intelligence. This powerful combination has the potential to transform operations, decision-making, and customer experiences across a wide range of domains. Let's explore these industries and sectors in detail:
1. Healthcare:
Big data analysis is revolutionizing healthcare by enabling predictive analytics, personalized treatment plans, and disease outbreak prediction. AI-powered medical imaging and diagnostics assist in early disease detection and monitoring, improving patient outcomes.
2. Finance:
In the financial sector, big data analysis and AI are used for fraud detection, risk assessment, and algorithmic trading. These technologies enhance customer experience through personalized financial recommendations and investment strategies.
3. Retail and E-Commerce:
Retailers employ big data analysis to optimize inventory management, pricing strategies, and supply chain logistics. AI-driven recommendation engines provide customers with tailored product suggestions, increasing sales and customer satisfaction.
4. Manufacturing:
Manufacturing benefits from predictive maintenance, quality control, and supply chain optimization. AI-driven analytics help in reducing machine downtime and enhancing production efficiency.
5. Energy and Utilities:
In the energy sector, big data analysis is utilized for grid management, predictive maintenance of infrastructure, and energy consumption optimization. AI helps in identifying energy-saving opportunities and reducing operational costs.
6. Transportation and Logistics:
Logistics companies use big data and AI to optimize routes, manage fleets, and predict maintenance needs. This leads to cost savings and more efficient delivery operations.
7. Telecommunications:
Telecommunication companies employ big data analytics to monitor network performance, improve customer service, and detect potential network disruptions. AI chatbots enhance customer support and issue resolution.
8. Agriculture:
Agriculture benefits from big data analysis by optimizing crop management, irrigation, and resource allocation. AI-driven systems provide farmers with real-time insights for better decision-making.
9. Education:
In the education sector, big data analysis and AI aid in personalizing learning experiences. Adaptive learning platforms adjust coursework based on individual progress, improving student outcomes.
10. Marketing and Advertising:
Marketers utilize big data analysis and AI to enhance campaign targeting, customer segmentation, and ad optimization. These technologies improve the effectiveness of marketing efforts.
11. Government:
Governments leverage big data analysis for policy decision support, public safety, and urban planning. AI-driven systems assist in traffic management, emergency response, and public service delivery.
12. Environmental Monitoring:
Big data analysis is instrumental in monitoring and addressing environmental issues, such as climate change, pollution control, and natural disaster prediction. AI algorithms help in analyzing vast datasets and making informed decisions for environmental preservation.
13. Sports and Entertainment:
Sports teams and entertainment companies use big data analysis to optimize player performance, enhance fan engagement, and tailor content recommendations. AI-driven insights contribute to better game strategies and content curation.
14. Pharmaceuticals:
In the pharmaceutical industry, big data analysis and AI accelerate drug discovery, clinical trials, and patient data analysis. This results in more efficient research processes and improved drug development.
15. Real Estate:
Real estate firms employ big data and AI for property valuation, market analysis, and predictive maintenance for building facilities. These technologies aid in making informed investment decisions.
16. Insurance:
Insurance companies rely on big data analysis and AI to assess risk, process claims, and personalize insurance policies. This leads to more accurate underwriting and improved customer satisfaction.
17. Human Resources:
HR departments use big data analysis for talent acquisition, employee performance evaluation, and workforce planning. AI tools assist in identifying the right candidates and optimizing employee retention.
the integration of big data analysis and artificial intelligence has far-reaching implications across various industries and sectors. These technologies are driving innovation, improving efficiency, and enhancing decision-making processes. As more data becomes available, the potential for transformative insights and benefits in these domains continues to expand.
Future Challenges and Trends in Big Data Analysis via Artificial Intelligence
The future of big data analysis through artificial intelligence promises both significant challenges and exciting trends. Let's delve into the boring details of what lies ahead in this domain:
1. Data Privacy and Security:
As the volume of data continues to grow, ensuring data privacy and security will remain a paramount concern. Compliance with regulations such as GDPR will be critical. AI-driven methods for anonymization and encryption will be developed to protect sensitive information.
2. Data Integration:
With data coming from diverse sources in various formats, integrating and harmonizing these datasets will be an ongoing challenge. Future trends will focus on building more efficient data integration tools and platforms that streamline this process.
3. Scalability:
As data continues to grow exponentially, scalability will be a significant challenge. Scalable AI algorithms and infrastructure will be crucial to handle larger datasets and more complex computations.
4. Real-Time Analysis:
The demand for real-time data analysis will increase. Future trends will center on developing AI solutions that can process and analyze data in real-time, allowing businesses to make immediate decisions.
5. Ethical AI:
The ethical use of AI in big data analysis will be a critical concern. Addressing biases in algorithms, ensuring transparency, and adhering to ethical principles will become increasingly important in the future.
6. Talent Shortage:
There is a growing shortage of professionals skilled in big data analysis and AI. This trend is expected to continue, and organizations will need to invest in training and education programs for their employees.
7. Explainable AI:
Understanding the rationale behind AI-generated insights will be vital. The development of explainable AI models that can provide clear explanations for their decisions will be a significant trend.
8. Edge Computing:
With the rise of IoT devices, data processing at the edge will become more common. AI algorithms will be deployed on edge devices to analyze data locally, reducing latency and enhancing efficiency.
9. Automated Machine Learning (AutoML):
AutoML will become more prevalent, allowing non-experts to build and deploy machine learning models with ease. This trend will democratize AI and big data analysis.
10. Quantum Computing:
The potential of quantum computing to revolutionize big data analysis is a future trend. Quantum computers have the potential to solve complex problems at incredible speeds, transforming the field.
11. Federated Learning:
Privacy concerns will drive the adoption of federated learning, a technique that enables AI models to learn from decentralized data sources without centralizing the data itself.
12. Industry-Specific Solutions:
There will be a growing demand for industry-specific AI solutions tailored to the unique needs of various sectors, such as healthcare, finance, and manufacturing.
13. Autonomous Systems:
AI-driven autonomous systems will become more common. These systems can make real-time decisions without human intervention, leading to efficiency and cost savings.
14. Advanced Analytics:
Future trends in big data analysis will involve more advanced analytics techniques, including natural language processing, image recognition, and sentiment analysis, to extract deeper insights from unstructured data.
15. Interdisciplinary Collaboration:
Collaboration between data scientists, domain experts, and AI researchers will increase. This interdisciplinary approach will drive innovation and address complex problems effectively.
16. Data Governance:
With the growing importance of data, data governance practices will evolve. Organizations will establish robust data governance frameworks to ensure data quality, compliance, and accountability.
the future of big data analysis via artificial intelligence holds both challenges and exciting trends. As data continues to proliferate, addressing privacy, scalability, ethics, and the shortage of skilled professionals will be vital. Simultaneously, trends in real-time analysis, AI explainability, edge computing, and industry-specific solutions promise to revolutionize how organizations harness the power of big data. Adapting to these trends and challenges will be essential for businesses and researchers in this dynamic field.
In conclusion
big data analysis using artificial intelligence is not just an ambitious technology; it has become a reality that brings about a real transformation in various fields. By leveraging innovative methods and applications, we can maximize the potential of big data and use it for making informed and precise decisions. Big data analysis using artificial intelligence has the capability to change how businesses and organizations operate and improve the services we receive. This field represents a bright future that increasingly encourages us to research, innovate, and evolve to contribute to enhancing our lives and addressing significant challenges.
What's Your Reaction?
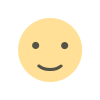
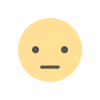
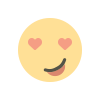
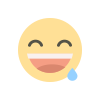
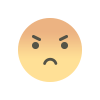
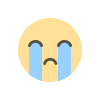
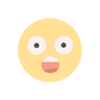